Machine Learning Spam Detection: Revolutionizing IT Services
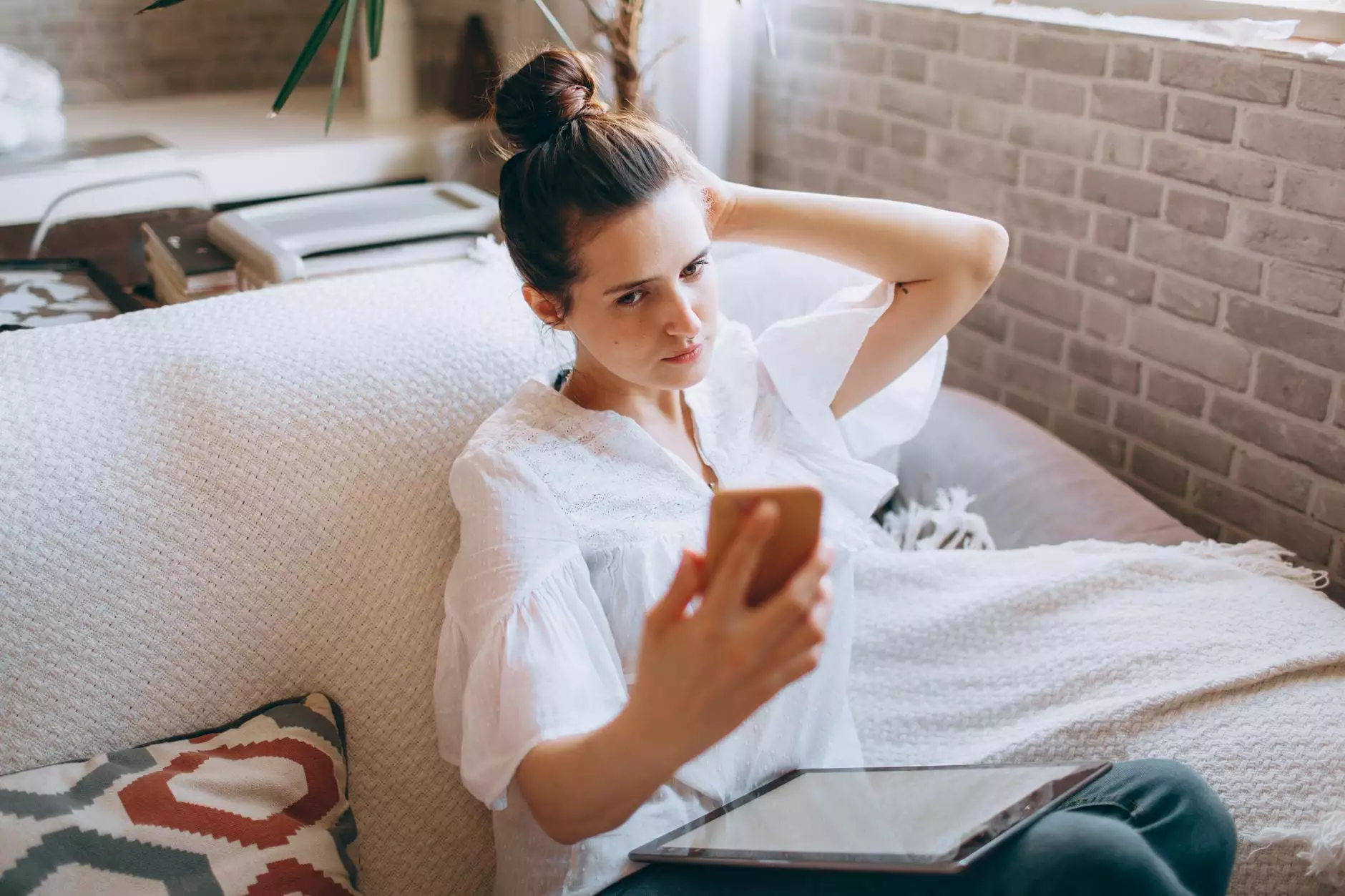
In today’s digital age, where the volume of email communications continues to rise exponentially, concerns regarding spam have become more relevant than ever. Spam emails, which clutter inboxes while often containing malicious links or unwanted advertisements, pose a significant threat to cybersecurity. Fortunately, machine learning spam detection is leading the charge in combating these nuisances in more sophisticated ways than traditional filtering methods.
Understanding Spam and Its Impact on Businesses
Spam not only irritates users but can also hinder productivity and jeopardize sensitive data. Here are some statistics to illustrate the impact of spam:
- According to studies, over 70% of all email sent is classified as spam.
- Businesses lose an estimated $20 billion annually due to spam-related issues.
- Cybercriminals often use spam emails as a vector for attacks, with more than 90% of malware being delivered via email.
As these figures illustrate, businesses need robust and adaptable strategies to manage spam effectively. This is where machine learning comes into play.
What is Machine Learning Spam Detection?
Machine learning (ML) is a subset of artificial intelligence that enables systems to analyze data, identify patterns, and make decisions with minimal human intervention. In the realm of spam detection, ML algorithms can learn from the characteristics of both spam and legitimate emails, continuously improving their accuracy over time.
Key Features of Machine Learning Spam Detection
Machine learning spam detection employs various techniques to enhance its efficacy. These include:
- Pattern Recognition: ML algorithms can identify patterns in email content, sender behavior, and metadata, enabling them to classify emails accurately.
- Adaptive Learning: Unlike standard filters, ML systems learn continuously, adapting to new spam tactics and updating their models accordingly.
- Natural Language Processing (NLP): NLP empowers ML models to comprehend and analyze the context of words and phrases, improving the filtering of phishing emails.
- Feedback Loops: User interactions with emails provide crucial data that helps refine the ML models, enhancing their performance over time.
Advantages of Implementing Machine Learning Spam Detection
Integrating machine learning spam detection into business processes offers numerous benefits:
- Increased Accuracy: ML-based systems significantly reduce false positives (legitimate emails marked as spam) and false negatives (spam that bypasses filters).
- Enhanced Security: By accurately identifying spam and phishing attempts, businesses can mitigate the risk of data breaches and cyberattacks.
- Time Efficiency: Employees can focus on their core tasks without the distraction of sorting through spam emails, thereby boosting productivity.
- Scalability: Machine learning systems can handle growing email volumes effortlessly, making them an excellent choice for businesses of all sizes.
Real-World Applications of Machine Learning Spam Detection
Many organizations across various sectors are leveraging machine learning spam detection to secure their communications:
1. Corporate Email Systems
Companies utilize ML algorithms in their email systems to filter spam effectively. Leading email providers, like Google Gmail and Microsoft Outlook, have already integrated these technologies to boost email management.
2. Customer Support Platforms
Customer support teams often face an influx of spam requests. By implementing ML spam detection, these teams can prioritize genuine customer inquiries, improving response times and customer satisfaction.
3. E-commerce Websites
E-commerce platforms receive numerous emails daily concerning orders and support. Machine learning helps ensure that only relevant communication reaches customer service representatives, creating a better shopping experience.
The Role of Security Systems in Machine Learning Spam Detection
As spam detection evolves, integrating ML with advanced security systems has become pivotal. Businesses can fortify their defenses against evolving spam tactics using these advanced technologies.
Enhanced Threat Detection
Security systems enabled with machine learning can detect harmful payloads within emails more efficiently. By analyzing data patterns and sender reputations, these systems can avert potential threats before they infiltrate corporate networks.
Combating Phishing Attacks
Phishing scams have become exceedingly sophisticated. Machine learning helps identify deceptive practices by analyzing irregular sender behavior and recognizing red flags in email content, keeping users safe from scams.
Challenges in Machine Learning Spam Detection
While machine learning spam detection offers numerous advantages, it is not without challenges:
- Data Quality: The effectiveness of machine learning algorithms largely relies on high-quality data. Poor or biased data can lead to inaccurate models.
- Resource Intensive: Training machine learning models can be computationally expensive and require significant resources, which may be a hurdle for smaller businesses.
- Evolving Spam Techniques: Spam techniques are continually evolving, requiring constant updates and retraining of models to stay effective.
Future Trends in Machine Learning Spam Detection
The landscape of email communication and spam detection is dynamic. Here are some trends likely to shape the future of machine learning spam detection:
1. Greater Integration with AI Technologies
As AI technologies continue to evolve, the capabilities of machine learning spam detection will expand. AI-driven analytics will provide deeper insights into email behaviors, resulting in even more refined models.
2. Increased Use of Big Data
Access to extensive datasets enables ML models to learn from a more diverse array of spam tactics, bolstering performance against sophisticated spam techniques.
3. Emphasis on Privacy
As privacy regulations become stricter, machine learning solutions will focus on protecting user data while effectively managing spam detection, ensuring compliance with laws such as GDPR.
Conclusion: The Future of Spam Detection in IT Services
As businesses continue to navigate the complexities of the digital environment, implementing effective spam detection strategies is critical. Machine learning spam detection offers a powerful tool for organizations like Spambrella.com in the IT Services & Computer Repair and Security Systems sectors, providing enhanced security and operational efficiency.
By adopting machine learning solutions, businesses can not only combat the challenges posed by spam and phishing attacks but can also improve overall productivity and foster a safer environment for their employees and clients. Embracing these innovations is no longer optional; it is a necessity for any organization wishing to thrive in the modern digital landscape.