Maximizing Efficiency with a Data Labeling Platform
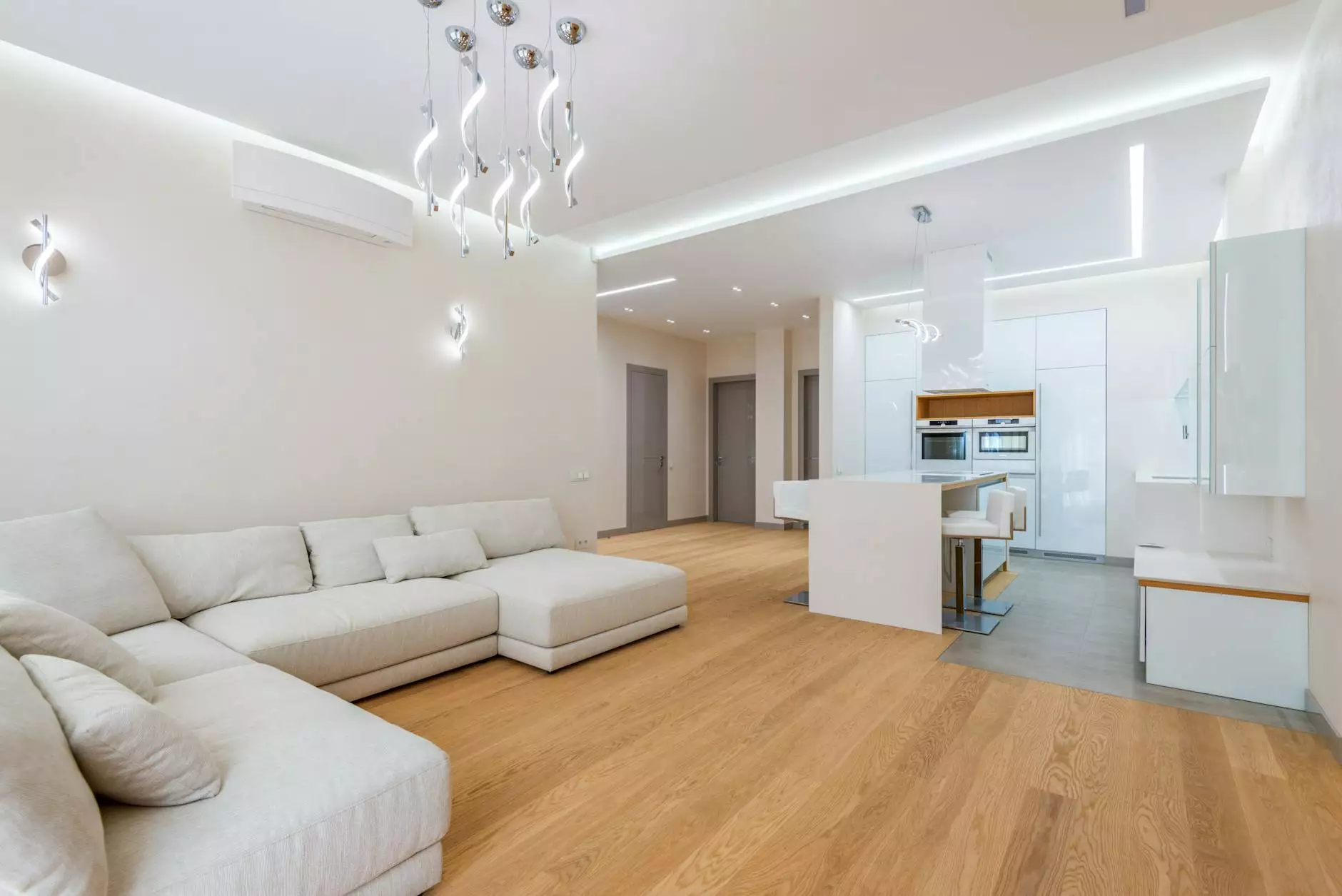
In the rapidly advancing world of artificial intelligence and machine learning, the importance of accurate data labeling cannot be overstated. A robust data labeling platform serves as the backbone for training sophisticated AI models, ensuring that they understand and interpret the vast amounts of information they process. In this article, we will explore the myriad benefits of utilizing a data labeling platform, the intricacies of the data labeling process, and how businesses can leverage it for unparalleled growth.
The Foundation of AI: Understanding Data Labeling
Data labeling is the process of tagging or annotating data to provide additional context that enables machine learning models to learn from that data effectively. This form of supervised learning is essential, as it allows algorithms to make predictions or classifications based on previously labeled inputs. Here are some key components of the data labeling process:
- Types of Data: Data labeling can be applied to various data types including text, images, audio, and video.
- Labeling Techniques: Techniques may vary from simple classification tasks to complex segmentation, where every pixel of an image is labeled.
- Quality Control: Ensuring the accuracy and consistency of labeled data is crucial for model performance.
Why Invest in a Data Labeling Platform?
Investing in a data labeling platform is not just a matter of convenience; it is a strategic decision that can propel your business forward. Here are some of the core benefits:
1. Enhanced Data Accuracy
A well-structured data labeling platform improves the accuracy of the labeled data. When data is accurately labeled, it enables the machine learning models to make informed predictions. This leads to not just better predictions but also an overall improvement in the model's trustworthiness.
2. Scalability
As your business grows, so does your data. A data labeling platform can easily scale to accommodate increasing volumes of data, allowing your organization to keep pace with the demands of machine learning applications without sacrificing quality.
3. Cost-Effectiveness
While many businesses may hesitate to invest in a data labeling platform due to initial costs, the long-term savings are significant. By streamlining the labeling process, organizations can reduce the time spent on data preparation, allowing for quicker deployment of models and faster iteration cycles.
Key Features of an Effective Data Labeling Platform
To maximize the potential of a data labeling platform, it’s critical to understand the features that make one effective. Here are the essential features to look for:
- Intuitive User Interface: A simple and user-friendly interface is vital to allow labelers to focus on their tasks without facing technical challenges.
- Collaboration Tools: Features that facilitate team collaboration enhance productivity and enable multiple teams to work on data labeling simultaneously.
- Quality Assurance Mechanisms: Built-in quality control features help maintain high standards by incorporating double-checks and feedback loops.
- Integration Capabilities: The ability to integrate with existing software solutions and data pipelines boosts efficiency and simplifies workflows.
Best Practices for Using a Data Labeling Platform
To get the most out of your data labeling platform, consider the following best practices:
1. Define Clear Labeling Guidelines
Establishing clear and concise labeling guidelines is crucial for consistency. These guidelines should be accessible to all team members involved in the labeling process, ensuring everyone is on the same page regarding expectations.
2. Invest in Training
Effective training for labelers is essential. Ensure that your team understands the nuances of the data they are labeling and the impact that quality labeling has on model performance.
3. Monitor and Improve
Constantly monitor the performance of your labeling efforts. Use metrics to assess accuracy and efficiency, and adjust your processes as necessary to ensure continuous improvement.
Real-World Applications of Data Labeling Platforms
Data labeling platforms are at the heart of various applications across multiple industries. Some notable examples include:
1. Autonomous Vehicles
In the automotive industry, data labeling is crucial for training self-driving car systems. High-quality labeling of images and sensor data ensures that vehicles can accurately recognize pedestrians, traffic signs, and other vehicles.
2. Healthcare
Healthcare organizations utilize data labeling to enhance diagnostic imaging applications. Effective labeling of X-rays, MRIs, and CT scans allows machine learning models to assist in identifying diseases and anomalies.
3. E-commerce
In retail, data labeling helps in categorizing products, analyzing customer reviews, and improving recommendation systems. Accurate labeling of product images and textual data enhances user experiences and increases sales.
Challenges in Data Labeling and How to Overcome Them
While data labeling is fundamentally important, it does come with its challenges. Here are some common obstacles and strategies for overcoming them:
1. Volume of Data
The sheer volume of data can be overwhelming. To tackle this, businesses can leverage automated tools within a data labeling platform to assist in initial tagging, allowing human resources to focus on more complex labeling tasks.
2. Subjectivity in Labeling
Different labelers may interpret labeling guidelines differently, leading to inconsistencies. Developing a robust training program and continually recalibrating guidelines can help mitigate this issue.
3. Time Constraints
Rushed labeling can lead to lower quality. Ensure that labeling tasks are distributed reasonably among team members, and prioritize quality over speed, especially during critical phases of model training.
The Future of Data Labeling Platforms
The landscape of data labeling is ever-evolving, driven by technological advancements and the growing needs of AI applications. The future holds exciting potentials:
- Increased Automation: More sophisticated automation tools will emerge to assist in labeling processes, reducing the burden on human labelers and speeding up data preparation.
- Enhanced AI Collaboration: Future platforms may employ AI to not only assist but also improve labeling accuracy based on learned data characteristics.
- Cross-industry Applications: As industries increasingly realize the value of data labeling, we can expect to see broader applications in sectors like finance, logistics, and education.
Conclusion
In conclusion, the data labeling platform is an indispensable tool in the software development landscape, especially in the realm of AI and machine learning. By investing in a robust platform, organizations can ensure they manage their data effectively, leading to better-trained models and enhanced overall performance. Embracing the best practices outlined in this article will not only enhance the quality of data labeling efforts but also empower businesses to harness the full potential of their data.
As the demand for machine learning and AI continues to rise, the importance of strategic investment in data labeling processes cannot be overlooked. Leverage these insights to create a competitive advantage and position your organization as a leader in the industry.